On Student Facing AI, Eduaide.Ai's Approach, and Transactional Alignment
- Date
- March 20, 2024
- Thomas Thompson
In educational technology, deliberate caution is a virtue—especially when discussing the integration of Artificial Intelligence (AI) into schools. Generative AI is developing rapidly and becoming ubiquitous as it's ingrained in our daily technological experiences. This rapidity of development generates considerable excitement and scorn. These competing reactions give rise to what we may describe as hype, which often features irrational exuberance and immovable cynicism. Both of these impulses are generally unproductive, which is put into stark relief when we consider the role of educational institutions. These institutions are not only centers of learning; they are custodians of public trust. They safeguard sensitive student data and academic information, all while carrying the significant responsibility of delivering high-quality education.1 As such, any technology introduced into this environment demands a thorough evaluation. Therefore, we must set aside hype and think deeply about use cases, the technology's capabilities, and the boundaries beyond which its effectiveness in aiding education diminishes. This is a standard of thought that we strive for when building Eduaide. It means stripping away all technology and asking fundamentally, what problems do we want to solve?
Challenges and Considerations for Implementing Student-Facing AI
One of the latest areas of concern is the implementation of student-facing AI. It is essential to establish robust guardrails before such a technology can be deemed ready for classroom use. Such safeguards must ensure that AI is constrained and operates within carefully defined educational parameters, promoting beneficial teaching and learning outcomes without compromising academic integrity, privacy, or stepping beyond the purview of the school's public function. These concerns are only compounded by the broader challenges of hallucinations and bias in AI response, as well as the practical considerations schools face when implementing new technologies—infrastructure requirements, teacher training, and the digital divide among students. Additionally, rigorous, unbiased research is needed to assess whether AI tools like Eduaide genuinely enhance learning and teaching processes before student-facing AI can be considered for classroom implementation.
Let's take some examples of student-facing AI—chatbots for students, fully automated feedback engines, LLM-based tutors—and run some thought experiments.
- Hallucinations: A hallucination is when an AI generates factually incorrect information. This may be a shortcoming of the architecture of LLMs and probability-based prediction—how the model selects the next word based on what it has learned from its training data. However, this probabilistic approach does not guarantee complete factual accuracy and can lead to hallucinations.2 In other words, responses or details in responses that are statistically plausible but factually incorrect. Additionally, imperfect training data and ambiguous prompting may also increase the likelihood of hallucinations.
Example: A student chats with an AI representation of a historical figure as part of a Social Studies lesson. The teacher does not moderate the discussion or serve as an intermediary. The chatbot provides incorrect biographical or historical information or misrepresents the individual. This can create stubborn misunderstandings where the student engages with false information during instruction, which becomes engrained in their prior knowledge as the course develops.
- Misuse: There is a need for robust content moderation guardrails on student-facing AI.
Example: A student gives a chatbot a particular persona that enables them to query it regarding illegal, unsafe, or inappropriate content. Without proper content moderation and guardrails, the AI might provide responses that reinforce harmful behaviors, offer suggestions for pursuing illicit activities, or expose the student to inappropriate content. This could have severe implications for the student's safety, well-being, and moral development.
- Bias and Discrimination: AI systems can perpetuate the biases in their training data.
Example: An AI-based grading system might evaluate essays in a way that favors particular dialects, language structures, or cultural references over others. This would disadvantage students from diverse linguistic and cultural backgrounds and could lead to unintentional yet systemic biases in grading. The teacher must be the intermediary who ultimately delivers feedback to the student. Building trust by providing students with timely, relevant, and actionable feedback is an aid to learning. It is sensible to create a system that heightens that teacher/student relationship rather than replace it.
- Privacy Concerns: Student-facing AI can collect, analyze, and store vast amounts of personal information, including academic records, performance results, and other potentially sensitive data. This raises significant privacy concerns, particularly regarding who has access to this data and how it is used.
Example: An AI learning platform collects detailed data on students' learning habits, strengths, weaknesses, and interests. If this data is not adequately protected and anonymized, it could be accessed by unauthorized parties, leading to privacy breaches. Additionally, if an AI's data is used to make decisions about the student without public transparency or measures for human oversight, it could lead to an erosion of the public trust in educational institutions as stewards of student data.
- Access: When implementing student-facing AI, the digital divide should be considered. Students from under-resourced communities may have limited access to the necessary technology, leading to unequal learning opportunities and exacerbating existing educational inequities.
Example: A school district introduces an AI-based personalized learning platform that requires high-speed internet and modern devices. Students from low-income families who lack access to such technology cannot participate equally, leading to a widening gap in educational outcomes between them and their more affluent peers.
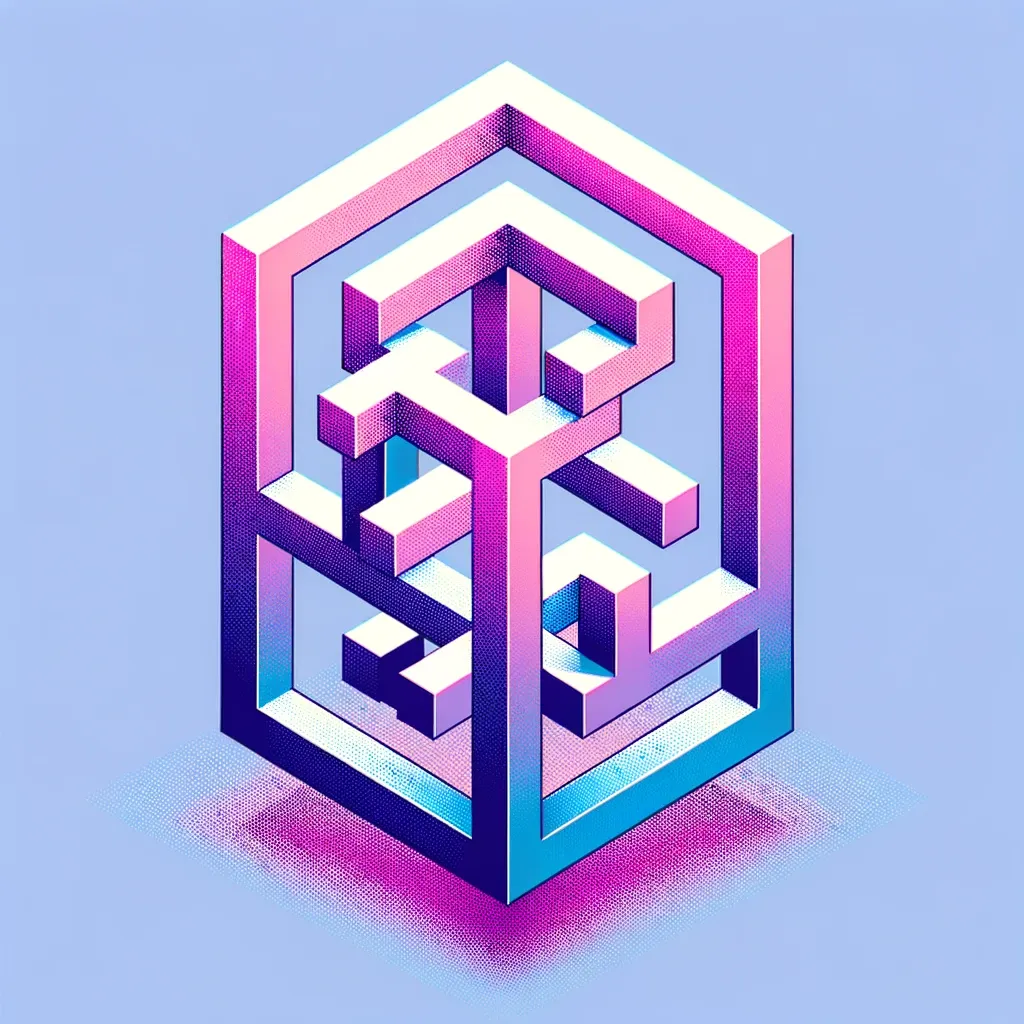
Eduaide's Approach: Teacher-Facing AI and Transactional Alignment
Navigating complexities like these is central to what we do at Eduaide. We concentrate on teacher-facing AI—building a robust workspace for instructional design that uses generative AI to integrate the variety of tools needed to plan a lesson in one place. In other words, AI can be used as tooling in the instructional design workflow, similar to what the Adobe suite offers for the photography or illustration workflows. This necessarily means that our solution is tightly aligned with educational objectives. Our development process acknowledges the pressing questions surrounding the effective constriction of AI in education and what ''alignment'' truly means within this context. Our approach to this challenge is centered on achieving transactional alignment, where the end-user—the teacher—creates what they need to enhance their instructional capabilities.3 That is, it places the teacher in the loop as an individual who has ownership over their classroom and will work with AI to more effectively and efficiently bring their instructional intentions to the students. Eduaide.Ai, in other words, is not meant to reinvent the teacher-student relationship but to augment it by creating many small efficiencies in the learning design process that add up to meaningful change.
To realize this goal, we employ several strategies:
- Modularity of Design: The application's user experience emphasizes stacking and combining resources to build a logical instructional sequence. In other words, each AI-performed task is broken down into fundamental components. Instead of generating a test, for example, Eduaide will generate possible assessment items that the teacher picks from to add to the assessment.
Throughout the application, the user must make deliberate choices about which components they require and how those components relate to their instructional objectives. This creates a human-in-the-loop environment in which the teacher collaborates with the AI within specific instructional parameters.
Granular Control: Eduaide ensures that all AI-generated content is editable. Teachers can modify, add to, or remix the generated material, offering them a sense of ownership over the content they present to their students.
Retrieval Augmented Generation: By grounding generations within a specific context by first retrieving relevant information from provided documents and then using that information to inform the generation process, we aim to minimize bias and inaccuracies in the generated material.
- Model Garden Approach & Prompt Design: We designed our platform with a variety of large language models (LLMs) in mind. This enables us to provide teachers with various tools, each selected for its unique functional capabilities.
- A Suite of Personalization and Differentiation Tools: Meeting learners' unique needs in a variety of instructional contexts requires some degree of personalization. With tools to chunk texts, adjust reading levels, inject prior knowledge scaffolding, language supports, or vocabulary development, Eduaide.Ai enables the teacher to further fine-tune generative outputs to meet their instructional goals.
Beyond the technical aspects, we also prioritize considerations of ownership and governance of AI in education, advocating for robust checks and balances that safeguard the interests of all involved, especially students. This consideration significantly affects our development roadmap as we conceive of what a user community will look like. How sharing, retaining, revising, remixing, and redistributing resources between teachers can create communities of practice. This collective intelligence can check the work of the individual intelligence of the teacher, augmented with the use of AI for instructional planning.
Integrating AI in education is undoubtedly complex and fraught with challenges. However, by adopting a deliberate, thoughtful, research-driven approach, we can better assess the potential of these technologies to refine how we approach teaching and learning.4 At Eduaide, we are optimistic about the future of AI in education. The space is filled with great companies and organizations working toward common aims. We believe that with ongoing research, iterative design, broad collaboration, open access, and a commitment to ethical standards and transactional alignment, we can ensure that AI becomes a valuable ally in our mission to provide high-quality education for all.
1. "trust is dependent upon the preservation of privacy and confidentiality" (Barquin & Northouse, 2003, p. 6). Relevant legal frameworks and guidance documents to privacy and education: PPRA (2020), FERPA (2011), COPPA (1998), HIPAA (1996), and applicable state laws addressing Student Data collection (E.g., New York Education Law §2-d).
2. See, Rawte et al., 2023; Ye et al., 2023; Lee, 2023
3. For a clearer sense of what we mean by Transactional Alignment, see Berg et al., 2023; Ganguli et al., 2023
5. Relevant literature and posts on frameworks for evaluating AI in education: Chan, 2023; Wiley, 2023; Chaudhry et al., 2022; Xu & Ouyang, 2022